What are Correlation Coefficients?
The ultimate guide to correlation coefficients
In this article
- What are Correlation Coefficients?
- What is a correlational study?
- What is a positive correlation?
- What is a negative correlation?
- When there is no correlation
- Correlational Study Designs
- Correlation does not equal causation
- Dynamic correlation
- Helpful Resources
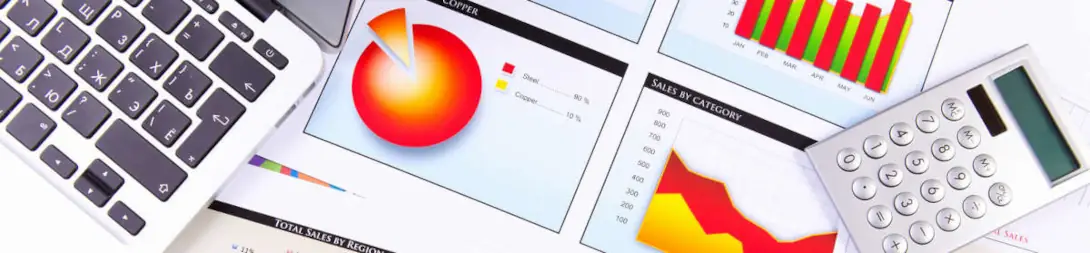
A correlation coefficient is the statistical measure that will tell us whether there is a relationship between our two variables of interest, and if there is one, how strong that relationship is. The value of the correlation coefficient, ρ (rho), ranges from -1 to +1. The closer to -1 or +1, the stronger the relationship is. We've also prepared a guide to nominal, ordinal, interval, ratio scales.
What is a correlational study?
The purpose of a correlational study is to examine the potential relationship between two variables. In this type of study design, researchers will quantify two variables of relevance to their research question, and then statistically determine if the two variables are related to one another.
For a correlational study, we may ask research questions such as:
- Is there a relationship between the number of cigarettes smoked per day and the likelihood of developing lung cancer?
- Is there a relationship between the number of hours spent exercising per week and levels of depression?
- Is there a relationship between the color of your shirt and the score you receive on a mathematics exam?
When to Use Correlational Research
Understanding the relationship between two or more variables is at the heart of correlational research. It’s a crucial method used in psychology, education, social sciences, and many other fields to explore connections that can lead to further investigation and discovery. Let’s dive into when this type of research is most effective and how it can be applied in real-world situations.
Exploring Relationships Without Manipulation
Use correlational research when you’re interested in discovering or examining the relationship between variables without altering them. This method observes variables as they are in real life, providing insights into how they coexist and affect each other.
Pre-experimental Research
Before conducting experiments, correlational studies can help identify potential variables of interest. By revealing associations, researchers can formulate hypotheses that are more grounded in observed phenomena.
When Ethical or Practical Limitations Arise
In scenarios where experimental manipulation is unethical or impractical (e.g., studying the effect of smoking on health), correlational research offers a viable alternative to explore relationships between variables.
Real-World Applications and Examples
- Education: Investigating the correlation between study habits and academic performance can help educators develop more effective teaching strategies.
- Health Sciences: Exploring the relationship between diet and health outcomes can inform public health recommendations.
- Psychology: Studying the connection between social media use and mental health can guide interventions to improve well-being.
Tips for Conducting correlational research
- Clearly define your variables and ensure they can be accurately measured.
- Use appropriate statistical methods to analyze the relationship between variables.
- Remember, correlation does not imply causation. Be cautious in drawing conclusions about the directionality of relationships.
How to collect correlational data
Correlational research offers a window into the intricate web of relationships between variables without necessitating experimental manipulation. This guide aims to provide a richer, more detailed exploration of the processes and considerations involved in gathering correlational data effectively.
Choosing Your Data Collection Method
Selecting a method to collect correlational data hinges on the research question, the nature of the variables involved, and the context of the study. Here are some refined strategies to consider:
Enhanced Survey Techniques
- Designing Questionnaires: Craft surveys with a mix of structured and open-ended questions to capture a broad spectrum of responses. Leveraging digital survey tools can enable sophisticated branching logic, ensuring respondents only see relevant questions, thereby increasing the accuracy of the data collected.
- Deploying Surveys: Use multi-modal distribution strategies (e.g., online platforms, social media, email campaigns) to reach a diverse audience. Ensure anonymity and confidentiality to improve response rates and honesty in answers.
- Sampling Strategy: Implement stratified random sampling or cluster sampling to ensure the sample is representative of the larger population, thereby increasing the external validity of the findings.
Refined Observation Techniques
- Setting and Context: Choose settings that are natural yet controlled enough to observe behaviors without significant external interference. Employ digital tools for recording observations to enhance precision.
- Quantitative Observation: Develop a standardized coding scheme for behaviors and phenomena of interest to ensure consistency and objectivity in data collection. Use time-sampling or event-sampling methods to systematically record observations.
Utilizing Secondary Data Sources
- Assessing Data Quality: Evaluate the credibility of the source, the methodology used to collect the data, and the relevance of the data to your research question. Adjust your analysis to account for any limitations in the secondary data.
- Integration of Multiple Data Sources: Combine data from various sources to enrich the dataset, cross-validate findings, and explore different dimensions of the research question.
Advanced Analytical Techniques
- Statistical Analysis: Beyond basic correlational coefficients, consider employing regression analysis to control for confounding variables, or factor analysis to identify underlying patterns in complex datasets.
- Interpreting Data: Carefully distinguish between correlation and causation. Utilize graphical representations (scatter plots, heat maps) to visualize relationships between variables.
Ethical Considerations
- Prioritize informed consent when using primary data sources.
- Ensure privacy and data protection, especially with sensitive information.
- When using secondary data, respect copyright and data usage policies.
Practical Examples
- Understanding Consumer Behavior: Analyze purchasing data and customer feedback surveys to identify patterns in consumer preferences and spending habits across different demographics.
- Environmental Studies: Correlate air quality data with health records to investigate the impact of pollution on respiratory health across different regions.
- Education Research: Examine the relationship between classroom technology use and student engagement by analyzing academic performance data and student surveys.
Collecting correlational data is a meticulous process that requires careful planning, ethical considerations, and sophisticated analysis. This exploration highlights advanced strategies and practical examples to guide researchers in effectively collecting and analyzing correlational data, paving the way for discoveries that deepen our understanding of the world around us.
How to analyze correlations
This guide delves into the essential steps and methodologies for analyzing correlational data, ensuring clarity, accuracy, and insight into the relationships between variables.
Step 1: Choose the Right Correlation Coefficient
Data Type | Correlation Coefficient | Use Case |
---|---|---|
Continuous Variables | Pearson's r | Measures linear relationship |
Ordinal Variables | Spearman's rho | Assesses monotonic relationships |
Mixed Variables | Point-biserial correlation | One continuous and one binary variable |
Tip: Ensure your data meets the assumptions (e.g., normality for Pearson's r) before selecting the coefficient.
Step 2: Visualizing Relationships
Creating scatterplots can help visualize the nature of the relationship between two variables. Scatterplots are invaluable for spotting patterns, trends, and potential outliers.
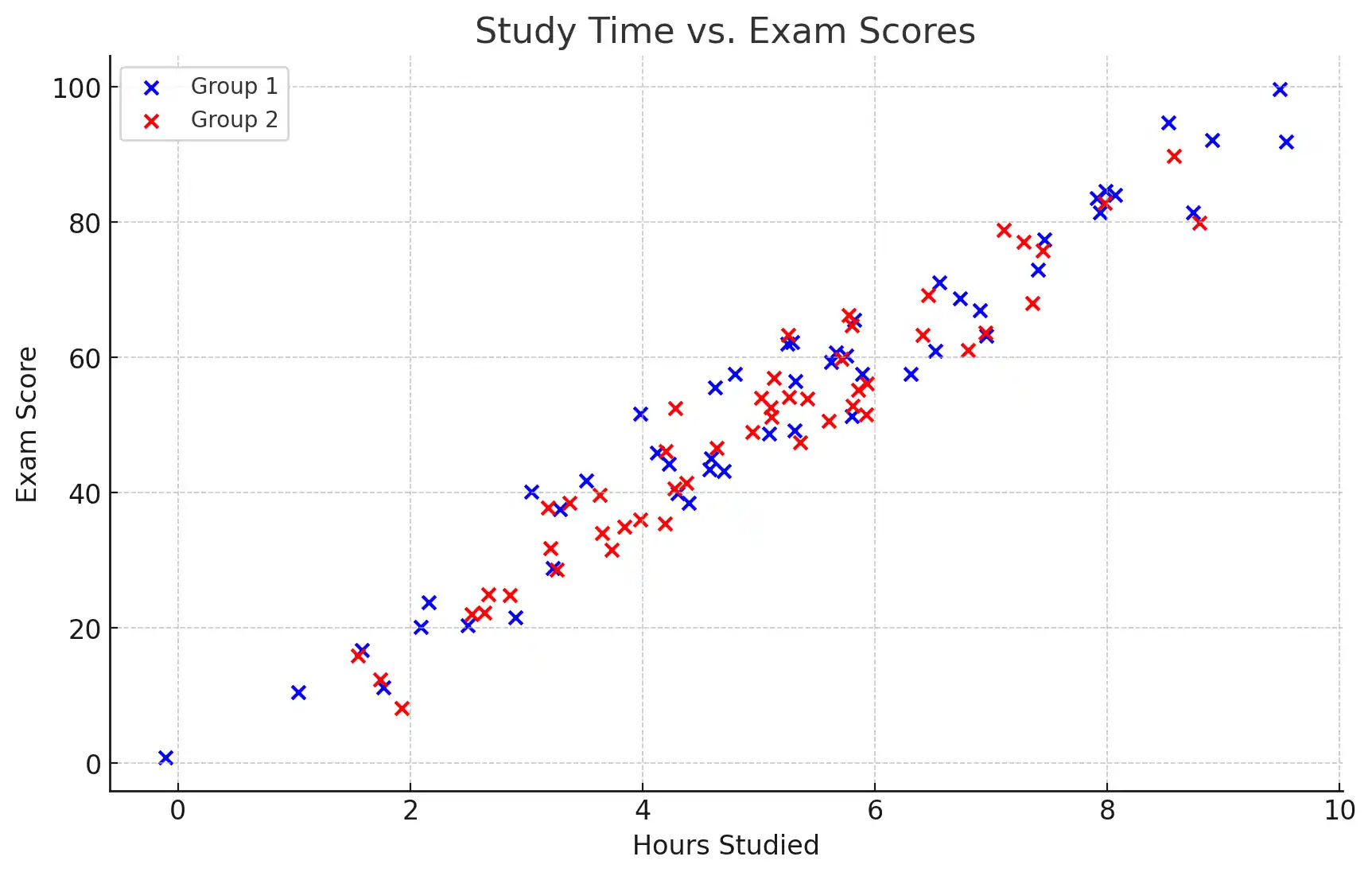
Visualization Tip: Use color coding or different symbols to represent different subgroups within your data, enhancing the interpretability of your scatterplot.
Step 3: Conducting the Analysis
Using statistical software, calculate the chosen correlation coefficient. A value close to +1 or -1 indicates a strong relationship, whereas a value near 0 suggests a weak relationship.
Example Output:
Pearson's r = 0.85 p-value < 0.001
This output indicates a strong positive relationship that is statistically significant.
Step 4: Interpreting Results
Interpretation goes beyond the numbers. Consider the context of your research, the strength and direction of the relationship, and its statistical significance.
Interpretation Tip: Discuss potential reasons for the observed relationship, but remember that correlation does not imply causation.
Step 5: Reporting Findings
When reporting your findings, include the correlation coefficient, its statistical significance, and a clear description of the relationship observed. Graphs and tables enhance readability and comprehension for your audience.
Armed with these steps and considerations, you’re well-equipped to analyze correlational data with confidence, providing insightful and reliable interpretations of the relationships within your data.
What is a positive correlation?
- Perfect Positive Correlation (in green): Shows a direct, flawless linear relationship where an increase in hours studied exactly predicts an increase in exam scores.
- High Positive Correlation (in blue): Demonstrates a strong but not perfect relationship, with some variability in exam scores even as hours studied increase.
- Low Positive Correlation (in red): Indicates a weaker relationship, where increases in hours studied have a less consistent effect on exam scores, shown by greater scatter.
When ρ is close to +1, this tells us that there is a positive, or direct, relationship between the two variables. This means that as one variable increases, the second variable also increases. Consider our first example: let's assume that there is a positive relationship between the number of cigarettes smoked per day and the likelihood of developing lung cancer. This means that as the number of cigarettes smoked per day increase, the chances of developing lung cancer also increase.
What is a negative correlation?
- Perfect Negative Correlation (in green): Demonstrates a precise inverse relationship, where an increase in hours studied results in a proportional decrease in exam scores.
- High Negative Correlation (in blue): Indicates a strong inverse relationship, with some variability in exam scores as hours studied increase.
- Low Negative Correlation (in red): Shows a weaker inverse relationship, with greater scatter and less predictability in how changes in hours studied affect exam scores.
- What our hypotheses are: if we wish to examine if there is a relationship between two variables, we need to base our predictions on existing research. For a correlation, we would therefore hypothesise that:
a. Null hypothesis: the correlation between the two variables is 0 (there is no relationship between the variables of interest); or
b. Alternate hypothesis: the correlation between the two variables is not 0 (there is a relationship between the variables of interest). - Inclusion of two quantitative and continuous variables: this means that both variables can have any numerical value, and should not be discrete (i.e. categorical). The two variables would be based on what relationship we want to look at.
- How we are measuring our variables: once we decide which variables we are investigating, we need to determine how they are going to be measured/quantified. How this is done depends on the variable of interest. For example, if we were measuring the number of hours of exercise, this could be a number recorded each day for a certain period. For depression levels, this could be quantified using a questionnaire. Another option is to use data that has already been collected (referred to as archival data). Determining a between or within-subjects design: we then need to decide who the variables are being measured in.This means that for our correlational study:
- JMP (2021). Correlations. https://www.jmp.com/en_au/statistics-knowledge-portal/what-is-correlation.html
- Australian Bureau of Statistics (2021). Statistical Language – Correlation and Causation. https://www.abs.gov.au/websitedbs/D3310114.nsf/home/statistical+language+-+correlation+and+causation
- Magnusson, K. (2020). Interpreting Correlations: An interactive visualization (Version 0.6.5) [Web App]. R Psychologist. https://rpsychologist.com/correlation/
In contrast, when ρ is close to -1, this tells us that there is a negative, or inverse, relationship between the two variables. This means that as one variable increases, the second variable decreases. Consider our second example: let's assume that there is an inverse relationship between the number of hours spent exercising and levels of depression. This means that as the number of hours spent exercising increases, levels of depression decrease. With a negative correlation, this could also be interpreted the opposite way: as the number of hours spent exercising decrease, levels of depression increase.
When there is no correlation
Alternatively, when ρ is close to 0, this means that there is a weak, or no, relationship between the two variables. This means that our two variables are likely not related to one another. Consider our third example: let's assume that there is no correlation between the color of your shirt and the score you receive on a mathematics exam. This means that whichever shirt you wear has nothing to do with how well you do on the test.
Correlational Study Designs
With a correlational study design, we wish to determine if there is a relationship between two variables. We therefore need to consider the following when designing our study:
a. Both variables should be measured on the same person or group of people (within-subjects); or
b. We have one variable measured in one group, and the other variable measured in another (between-subjects).
Once we have designed our correlational study, collected our data and calculated the correlation coefficient, we can then conclude if there is a relationship between our two variables, and if there is one, what kind of relationship it is (positive, negative, or none; and how strong that relationship is).
Correlation does not equal causation
It's important to remember that a correlation will only tell us if there is a relationship between our two variables. However, correlation does not equal causation. Based on a correlation, we cannot infer that one variable causes the change (increase or decrease) in the other, but we can only see that a relationship exists.
Dynamic Correlations
Another key factor is that correlations can be dynamic. A relationship between two variables may be present now, however it's not set in stone. For example, a positive correlation may become negative or zero in future studies due to a variety of factors, such as different sample sizes, measurement of the two variables in different groups (e.g. measuring them in the elderly instead of teenagers), measurement of the two variables using different approaches (e.g. changing questionnaires), and so on. This is what makes research interesting - seeing how relationships change!