Statistical Significance
Unlock the Secrets of Statistical Significance: Where Data Drives Decisions!
In this article
- What Does Statistical Significance Mean?
- One-Tailed and Two-Tailed Tests
- How to Calculate Statistical Significance
- Understanding the Likelihood of Error
- Helpful References
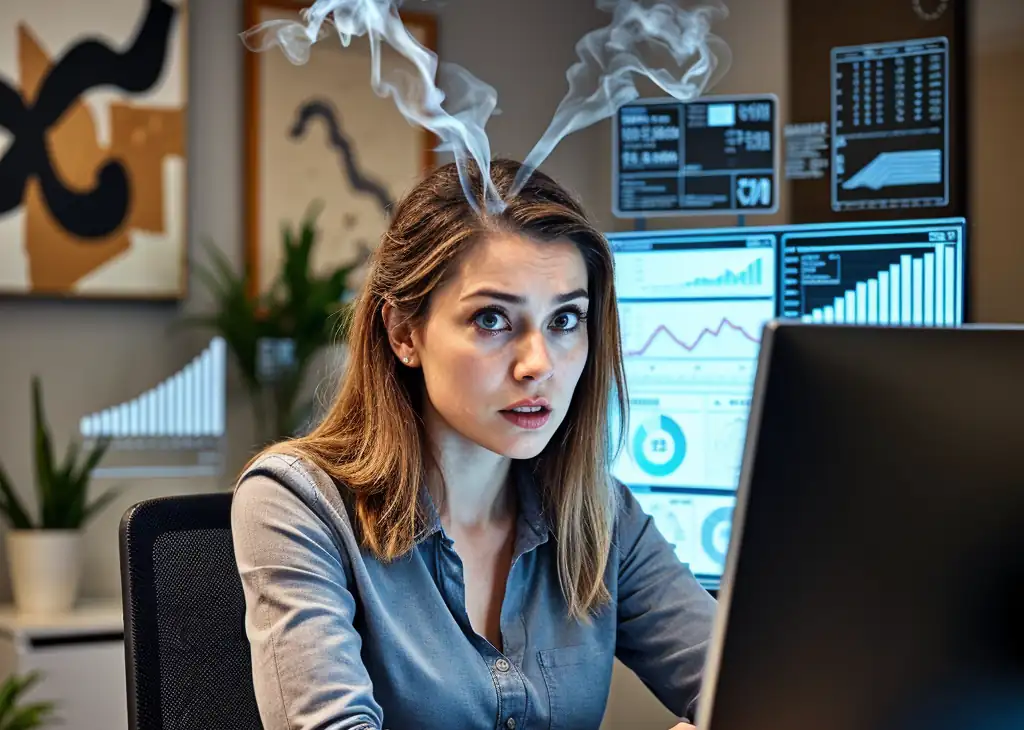
What Does "Statistical Significance" Really Mean?
The term "statistical significance" often pops up in research and data analysis, but what does it actually mean? At its core, statistical significance is a way to express how confident you can be that your findings are not just a fluke. It's like a stamp of reliability on your results, assuring you that what you're seeing is likely real.
However, it's important to note that statistical significance doesn't automatically imply that your findings are groundbreaking or of practical importance. Think of it as being sure that something happened, but not necessarily that it's something you need to act on. If you're interested in understanding how statistical significance fits into broader research practices, take a look at this guide on conducting effective research.
Let's swap out the typical examples and take a look at something more relatable. Imagine you're a coffee shop owner testing two new blends of coffee to see which one your customers prefer. You survey 500 people, and Blend A gets a thumbs up from 260 people, while Blend B is preferred by 240. You might think, "Blend A is the winner!" But hold on - before you start stocking up on Blend A, you run a statistical test and find that this difference is statistically significant at the 0.05 level. What does that really tell you?
What this means is that, given the sample size, you can be fairly confident that Blend A is genuinely preferred by your customers, and this result didn't just happen by chance. But here's the kicker: the difference in preference is small - just 20 votes out of 500. Is that really enough to overhaul your coffee lineup? Maybe, maybe not. The significance here mainly comes from the number of people surveyed. With a smaller group, this difference might not have been significant at all.
This brings us to a key point: statistical significance tells us that a difference or relationship exists, but it doesn't tell us how big or important that difference is. For example, if you had only asked 50 customers instead of 500, that 20-vote difference might not have shown up as significant, even if it's just as real.
In essence, statistical significance is a tool to help you decide whether your findings are worth paying attention to, but it's not the final word. After establishing significance, it's crucial to consider the magnitude and practical relevance of the result. A statistically significant finding can still be trivial in the grand scheme of things, depending on the context and your specific goals. If you're considering how this applies to sample sizes and their impact, check out this article on sample size determination.
There's also a common trap where "significant" is used interchangeably with "important." While this might work in everyday conversation, in the realm of statistics, "significant" has a very specific meaning - it refers to the likelihood that a result is not due to random chance. That said, in business and other decision-making environments, the term often takes on a broader meaning. This is why it's important to clearly distinguish between "statistical significance" and general "significance" when discussing your findings. For a deeper dive into interpreting data, you might find this resource on quantitative data analysis useful.
Example: Statistical Significance in Action
To further illustrate, let's consider an example in the realm of digital marketing. Suppose you're testing two versions of a landing page to see which one generates more leads. Here's what you find:
Landing Page | Visitors | Leads Generated |
---|---|---|
Version A | 8,000 | 600 |
Version B | 7,900 | 650 |
On the surface, Version B seems to be performing better with more leads. But to be confident that this difference is not just due to chance, you conduct a statistical test and find that the results are statistically significant. This tells you that the difference in performance between the two versions is likely real, and not just a result of random variability.
However, the next step is to consider the practical implications. Is a 50-lead difference over 8,000 visitors significant enough to justify switching to Version B? That's where you'll need to weigh the statistical significance against the practical relevance for your business objectives. For more information on how to approach this type of analysis, visit our regression analysis guide which helps you understand and predict outcomes.
Statistical significance is a powerful indicator in research, but it's just the beginning of a deeper analysis. By combining statistical insights with practical considerations, you can make informed decisions that truly make a difference.
Understanding One-Tailed and Two-Tailed Significance Tests
When diving into the world of statistical significance, one of the key decisions you'll make is whether to use a one-tailed or two-tailed test. The choice hinges on the nature of your hypothesis and what you aim to discover through your research.
If your hypothesis predicts not just that a difference or relationship exists, but also the direction of that difference, a one-tailed test is typically the way to go. For instance, suppose you're testing whether a new training program improves employee productivity. If you specifically hypothesize that the program will lead to higher productivity (and not lower), a one-tailed test will focus solely on this possibility. Examples of hypotheses that might call for a one-tailed test include:
- Men will not perform significantly better than women in a physical endurance test.
- Students who study in the morning will score higher on exams than those who study at night.
- A new marketing strategy will lead to increased sales compared to the old strategy.
In each case, the hypothesis anticipates a specific direction of the effect, making a one-tailed test a precise tool for testing that expectation.
On the other hand, if your hypothesis is neutral and simply tests whether there is any difference or relationship, regardless of direction, you'll want to use a two-tailed test. This is often the default choice in research when you want to ensure that your analysis captures any significant findings, whether they go in the expected direction or not. Here are examples of hypotheses suited for a two-tailed test:
- There will be no significant difference in academic performance between students attending online versus in-person classes.
- There is no significant difference in customer satisfaction between two different service methods.
- The average running speed of participants in two different training groups will not differ significantly.
The key distinction is that a one-tailed test is more focused, examining one specific direction, while a two-tailed test is more exploratory, open to detecting differences in either direction. A practical point to note is that a one-tailed test will yield a probability value that is exactly half of that from a two-tailed test, because it only looks at one side of the distribution. For more insights on testing strategies and their implications, see our sample size guide.
The Debate: One-Tailed vs. Two-Tailed Tests
There has been ongoing debate for over a century about the appropriateness of one-tailed tests. Critics argue that if you already expect a specific direction, why run the test at all? Proponents, however, point out that in certain cases, a one-tailed test is perfectly justified - especially when a specific outcome is of particular interest.
Ultimately, the choice between a one-tailed and two-tailed test often comes down to the specific goals of your research. While two-tailed tests are generally considered safer and more conservative, there are instances where a one-tailed test might be more aligned with your hypothesis and research objectives. The decision is yours as the researcher, but it's always good to be clear about your rationale and the implications of your choice.
How to Calculate Statistical Significance
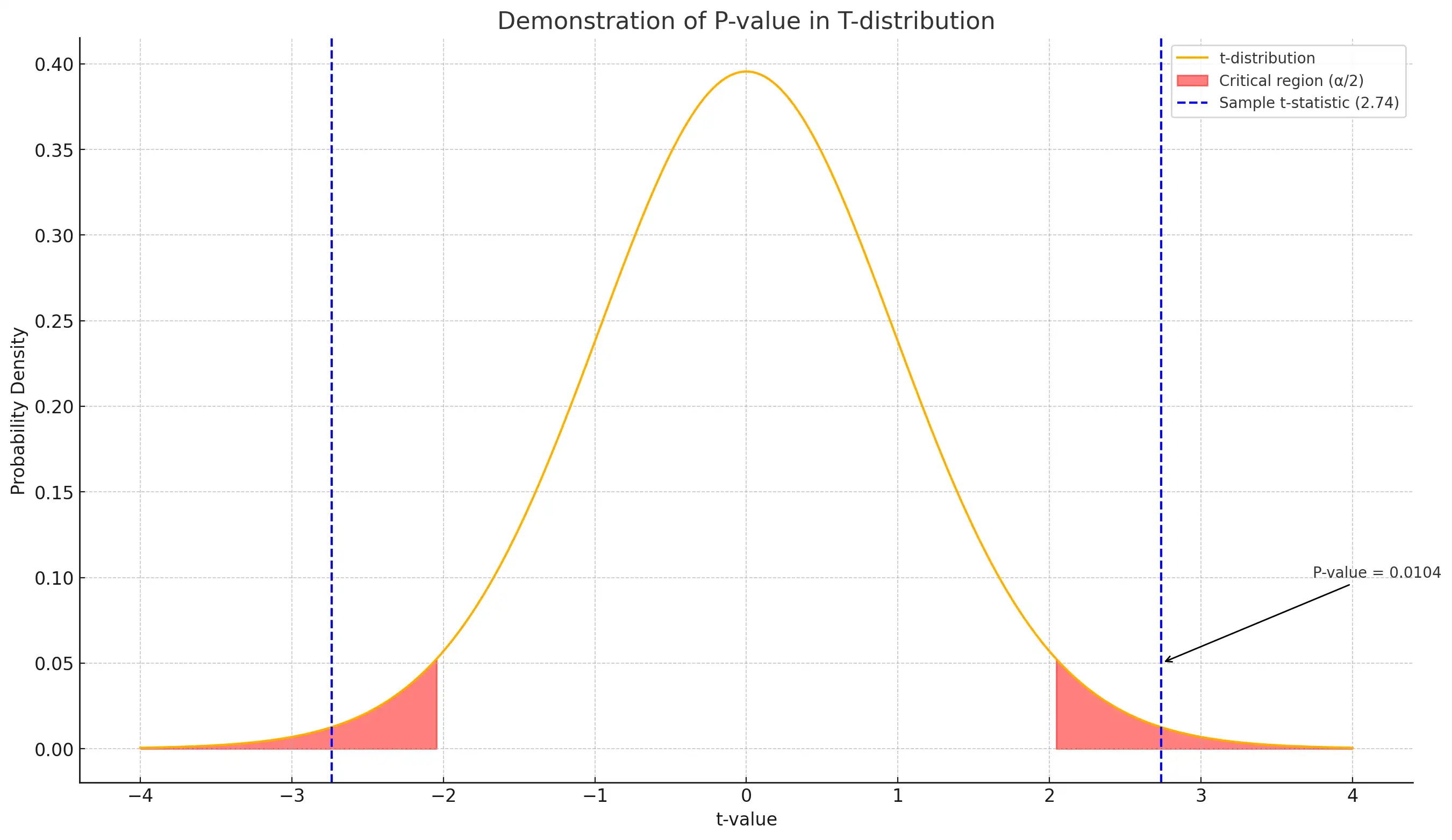
Statistical significance is a cornerstone of data analysis, helping researchers, analysts, and businesses alike determine whether the results of an experiment or study are meaningful or if they could have occurred by random chance. In this extensive guide, we will walk through every step involved in calculating statistical significance, from formulating hypotheses to interpreting results. By the end, you'll have a robust understanding of how to apply these concepts to your own data, ensuring that your conclusions are both reliable and actionable.
Understanding Statistical Significance
Before diving into the calculations, it's important to understand what statistical significance really means. In simple terms, statistical significance is a measure of whether an observed effect or relationship in your data is likely to be genuine or if it could have occurred by chance. It provides a level of confidence that the results you see are not random anomalies but are reflective of a real underlying pattern or difference. For a deeper dive into how this concept plays out in various research contexts, visit this comprehensive research guide.
Statistical significance is typically evaluated using a p-value, which is the probability of observing the data (or something more extreme) assuming the null hypothesis is true. The lower the p-value, the stronger the evidence against the null hypothesis. A result is generally considered statistically significant if the p-value is below a predetermined threshold, known as the alpha level, commonly set at 0.05.
1. Define Your Research Questions and Hypotheses
The first and arguably most important step in any statistical analysis is to clearly define your research questions and formulate your hypotheses. This involves specifying what you're trying to test and what you expect to find. If you're exploring how hypotheses shape the direction of research, our data analysis guide offers additional insights.
Null Hypothesis (H0)
Your null hypothesis should state that there is no effect or difference between the groups or variables you're comparing. For example, if you're testing a new drug, your null hypothesis might be that the drug has no effect on patient outcomes compared to a placebo.
Alternative Hypothesis (H1)
The alternative hypothesis is what you hope to prove. It suggests that there is a significant effect or difference. Continuing with the drug example, your alternative hypothesis might be that the drug improves patient outcomes compared to the placebo.
It's crucial to formulate these hypotheses clearly and concisely, as they guide the entire analytical process. They also determine whether you'll use a one-tailed or two-tailed test, which we'll discuss later.
2. Determine the Significance Level (Alpha)
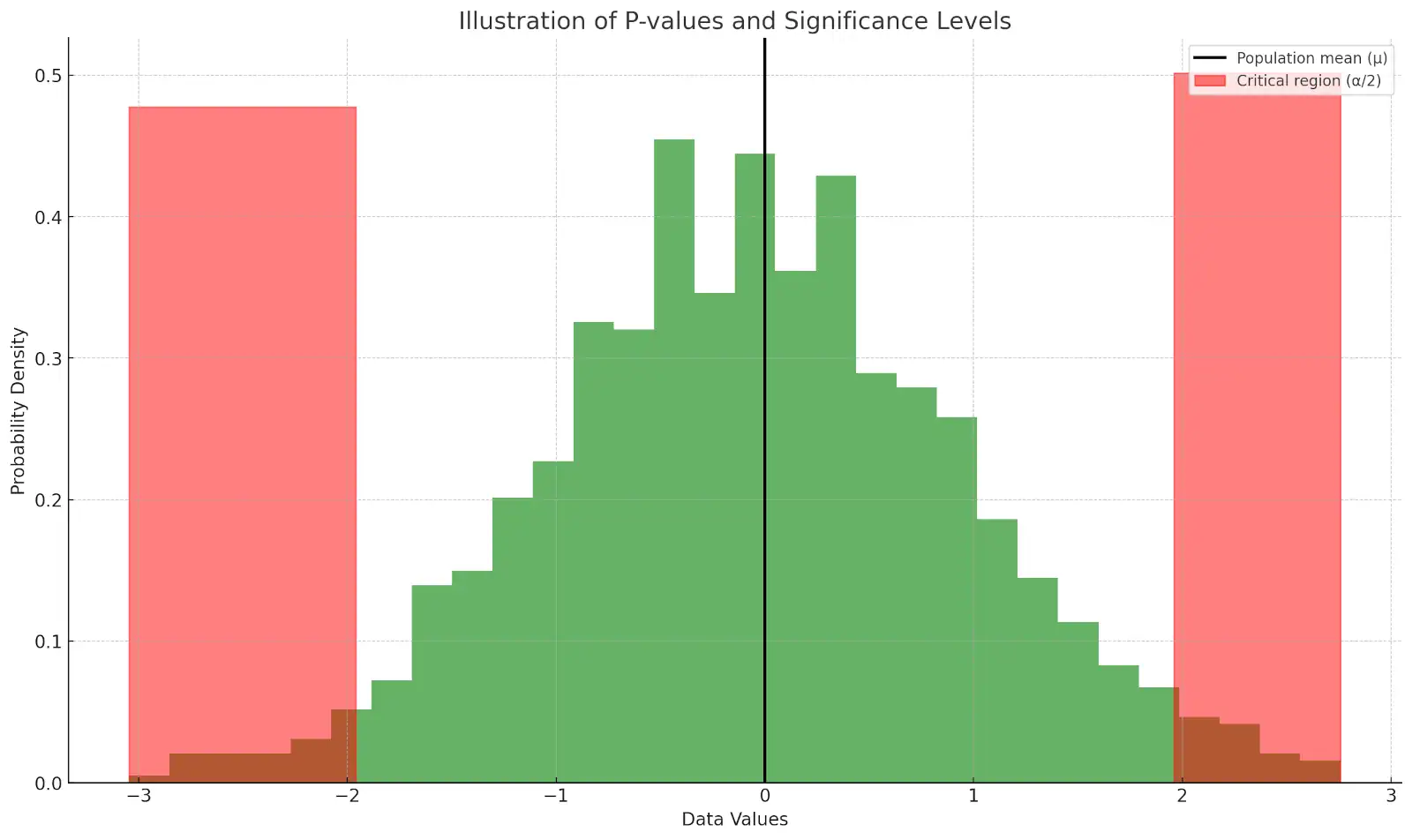
The significance level, often denoted as alpha (α), is the threshold at which you decide whether to reject the null hypothesis. The alpha level represents the probability of making a Type I error - rejecting the null hypothesis when it is actually true. Common alpha levels are 0.05 (5%) and 0.01 (1%), meaning you are willing to accept a 5% or 1% chance, respectively, of concluding that an effect exists when it does not. To explore more on the topic of errors and their implications, check out this detailed resource on Type I and Type II errors.
Choosing an appropriate alpha level depends on the context of your study. A lower alpha level (e.g., 0.01) requires stronger evidence to reject the null hypothesis, which is often used in fields like medicine where the cost of false positives is high. Conversely, a higher alpha level (e.g., 0.10) may be used in exploratory research where the consequences of Type I errors are less severe.
3. Choose the Appropriate Statistical Test
The choice of statistical test depends on your research design, the type of data you have, and the hypotheses you're testing. Some of the most commonly used statistical tests include:
- t-Test: Used to compare the means of two groups. There are two types: independent samples t-test (for comparing two different groups) and paired samples t-test (for comparing two related groups, such as the same group before and after treatment).
- ANOVA (Analysis of Variance): Used when comparing the means of three or more groups. It helps determine whether at least one group mean is different from the others. For more details on this method, explore our regression analysis guide.
- Chi-Square Test: Used for categorical data to assess whether there is an association between two variables.
- Regression Analysis: Used to explore the relationship between a dependent variable and one or more independent variables. It helps predict outcomes and assess the strength of relationships.
One-Tailed vs. Two-Tailed Tests
The decision to use a one-tailed or two-tailed test hinges on your hypothesis. A one-tailed test is used when you have a specific direction of interest (e.g., you believe one group will have a higher mean than another). A two-tailed test is used when you are looking for any significant difference, regardless of direction. For further reading on choosing the right statistical test, see our sample size and power analysis guide.
While a one-tailed test can be more powerful if your hypothesis is correct, it also carries a higher risk of Type I errors if the effect is in the opposite direction. Therefore, two-tailed tests are generally preferred unless you have a strong theoretical justification for a one-tailed test.
4. Conduct a Power Analysis to Determine Sample Size
A power analysis is a crucial step in the design phase of your study. It helps you determine the minimum sample size needed to detect an effect of a given size with a certain degree of confidence. The main components of a power analysis include:
- Effect Size: A measure of the magnitude of the effect you expect to find. Larger effect sizes require smaller sample sizes, while smaller effect sizes require larger samples to detect.
- Significance Level (Alpha): As discussed, this is the probability of rejecting the null hypothesis when it is true. Lower alpha levels require larger sample sizes.
- Power (1-β): The probability of correctly rejecting the null hypothesis when it is false. Commonly set at 0.80, meaning you have an 80% chance of detecting an effect if it exists.
- Sample Size: The number of participants or observations needed to achieve the desired power level.
Performing a power analysis ensures that your study is adequately powered to detect meaningful effects, reducing the likelihood of Type II errors (failing to detect an effect that is actually there). For more on the importance of sample size in research, refer to this detailed guide.
5. Collect and Organize Your Data
With your sample size determined, it's time to collect data. This step involves gathering all relevant information according to your research design. It's crucial to maintain consistency and accuracy during data collection to minimize bias and errors.
Once data collection is complete, organize your data in a structured format, such as a spreadsheet or database, making it easier to analyze. Ensure that all data points are properly labeled and that missing data is handled appropriately (e.g., through imputation or exclusion).
6. Calculate Descriptive Statistics
For more on handling and analyzing data, visit our data analysis guide, which offers detailed insights into various statistical techniques.
7. Calculate the Standard Deviation
Standard deviation is a key measure of variability in your data. It tells you how spread out the data points are from the mean. A high standard deviation indicates that the data points are widely dispersed, while a low standard deviation indicates that they are clustered closely around the mean.
To calculate standard deviation, use the following formula:
Standard Deviation (σ) = √((∑|x−μ|^2) / (N-1))
Where:
- ∑ = the sum of the data points
- x = individual data points
- μ = the mean of the data
- N = the total number of data points
This calculation provides a baseline understanding of the variability within your data, which is critical for interpreting the results of your significance tests. Understanding variability is essential in fields like research and marketing analysis, where precision is key to making informed decisions. For additional reading on this topic, visit this guide on statistical methods.
8. Apply the Standard Error Formula
The standard error is a measure of the precision of the sample mean as an estimate of the population mean. It accounts for both the variability within each group and the size of the sample. The smaller the standard error, the more reliable your estimate of the mean.
To calculate the standard error when comparing two groups, use the formula:
Standard Error (SE) = √((s12/N1) + (s22/N2))
Where:
- s1 = the standard deviation of the first group
- N1 = the sample size of the first group
- s2 = the standard deviation of the second group
- N2 = the sample size of the second group
This formula helps you understand the variability of the sample means, which is essential for determining the significance of your findings. Accurate calculations of standard error are crucial for reliable statistical analysis. To dive deeper into this topic, check out the research methodology guide.
9. Calculate the T-Score
The t-score measures the size of the difference relative to the variation in your sample data. It is used in hypothesis testing to determine whether to reject the null hypothesis. The formula for calculating the t-score is:
T-Score = (μ1 - μ2) / SE
Where:
- μ1 = the mean of the first group
- μ2 = the mean of the second group
- SE = the standard error
A higher t-score indicates a greater difference between the groups, which may suggest a significant result depending on the degrees of freedom and the critical value from the t-distribution. For those looking to refine their understanding of hypothesis testing, consider exploring additional resources on advanced statistical techniques.
10. Determine Degrees of Freedom
Degrees of freedom (df) are an essential part of calculating statistical significance. They refer to the number of values in the final calculation that are free to vary. The degrees of freedom for a two-sample t-test are calculated as:
Degrees of Freedom (df) = N1 + N2 - 2
Where:
- N1 = the sample size of the first group
- N2 = the sample size of the second group
Degrees of freedom help you determine the critical t-value from the t-distribution table, which is used to assess the significance of your t-score. For further exploration of how degrees of freedom impact statistical testing, refer to our sample size guide.
11. Use a T-Table to Determine Statistical Significance
The final step in calculating statistical significance involves using a t-table. The t-table provides critical values for the t-distribution, which you can compare with your calculated t-score. Here's how to use it:
- Find your calculated t-score.
- Identify the degrees of freedom (df) for your test.
- Look up the critical value in the t-table corresponding to your alpha level (e.g., 0.05) and degrees of freedom.
- Compare your t-score to the critical value:
If your t-score is greater than the critical value, you reject the null hypothesis, indicating that the difference between groups is statistically significant. If your t-score is less than the critical value, you fail to reject the null hypothesis, meaning there is no significant difference.
Example: Comparing Marketing Strategies Across Two Regions
Let's say you are a marketing analyst comparing the effectiveness of two different marketing strategies across two regions. Here's a practical example of how you might calculate statistical significance using the steps outlined above:
You've collected data on sales from Region A and Region B over a six-month period. Your hypothesis is that the new marketing strategy implemented in Region A leads to higher average sales compared to Region B, which used the traditional strategy.
Region | Number of Sales | Average Sale Value | Standard Deviation |
---|---|---|---|
Region A | 1,000 | $120,000 | $15,000 |
Region B | 950 | $115,000 | $14,500 |
Using the formulas and steps provided earlier, you would calculate the t-score, degrees of freedom, and finally determine the statistical significance using a t-table. If your analysis shows a t-score greater than the critical value from the t-table, you can conclude that the new marketing strategy in Region A has a statistically significant impact on sales compared to Region B.
Reporting Your Findings
Once you've completed your analysis, it's essential to report your findings in a clear and concise manner. When presenting your results to stakeholders, include the following:
- Summary of Findings: Clearly state whether the results were statistically significant and what that means for your research question or business decision.
- Context and Implications: Discuss the practical implications of your findings. If the difference is statistically significant, explain how this will impact future strategies or decisions.
- Limitations: Acknowledge any limitations in your study, such as sample size or potential biases, and suggest areas for further research.
- Next Steps: Provide recommendations based on your findings, whether that involves implementing a new strategy, conducting additional tests, or re-evaluating your hypotheses.
Conclusion: The Importance of Understanding Statistical Significance
Calculating statistical significance is a fundamental skill in data analysis, enabling you to distinguish between real effects and random variation. By following the steps outlined in this guide, you can confidently analyze your data, interpret the results, and make informed decisions that are grounded in statistical evidence.
Remember, while statistical significance is a powerful tool, it's not the final word. Always consider the practical significance of your findings and how they fit into the broader context of your research or business goals. Practical significance involves looking beyond the p-value to understand the real-world impact of your results. For instance, even if a result is statistically significant, it might not be meaningful for your business strategy if the effect size is too small to justify a change in direction. For more insights on this topic, consider reading up on regression analysis and its applications in business decision-making.
With a solid understanding of these concepts, you're well-equipped to turn data into actionable insights. Whether you're conducting academic research, evaluating marketing strategies, or making business decisions, understanding and correctly applying statistical significance can significantly enhance the quality of your conclusions and recommendations.
Understanding the Likelihood of Error
When working with data and statistics, there's always a chance that errors can creep into your results. It's important to be aware of these potential pitfalls, especially when you're interpreting statistical significance. Two common types of errors to watch out for are Type I and Type II errors.
Type I Error (False Positive): This error occurs when you reject the null hypothesis even though it's actually true. In simpler terms, you're finding a significant result where there isn't one. It's like a false alarm - your data might suggest a difference or effect, but it's actually just due to random chance. In the real world, this could mean acting on findings that don't really hold up under further scrutiny.
Type II Error (False Negative): On the flip side, a Type II error happens when you fail to reject the null hypothesis when it's actually false. This means you're missing a real effect or difference because the data didn't show it as significant. It's as if you overlooked something important that's actually happening. In practical terms, this could lead to missing out on valuable insights or opportunities because your analysis didn't catch the significant result.
Being aware of these errors helps you navigate the complexities of statistical analysis more confidently, ensuring that your conclusions are both accurate and reliable.
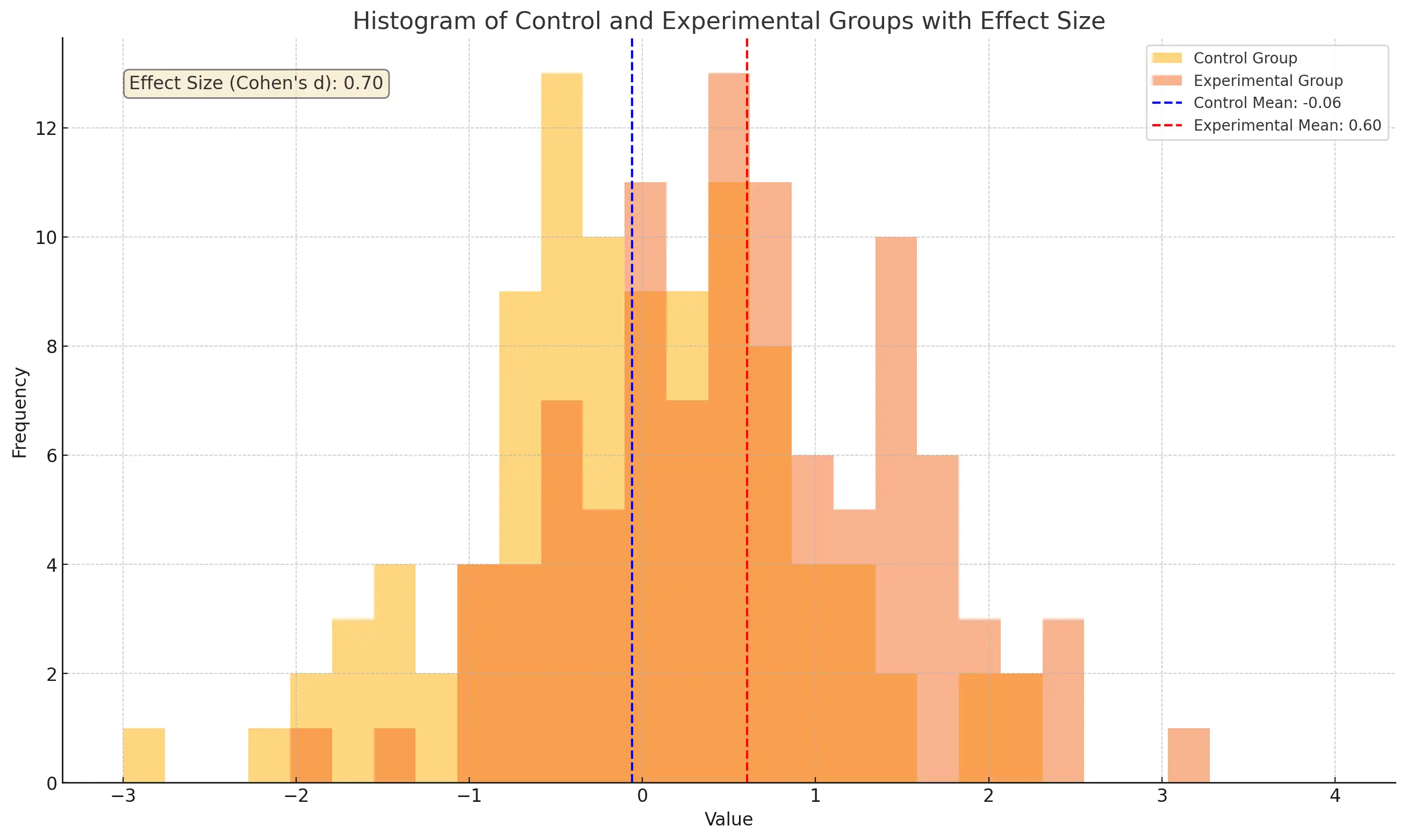
Just because a result has statistical significance, it doesn't mean that the result has any real-world importance. To help 'translate' the result to the real world, we can use an effect size. An effect size is a numerical index of how much your dependent variable of interest is affected by the independent variable, and determines whether the observed effect is important enough to translate to the real world.Therefore, effect sizes should be interpreted alongside your significance results.The two main types of effect size include Cohen's d, which indexes the size of the difference between two groups in units of standard deviation. For Cohen's d, a score of 0.2 is a small effect, 0.5 is a medium effect, and 0.8 is a large effect. The other effect size is eta-squared, with measures the strength of the relationship between two variables. For eta-squared, a score of .05 is a weak effect, .10 is a medium effect, and .15 is a strong effect. Both of these effect sizes can be calculated by hand, or you can ask for it to be calculated for you as part of statistics software.
Make a Free SurveyHelpful References:
- Australian Bureau of Statistics (2011). Significance Testing.
- Statistics by Jim - Understanding Significance Levels in Hypothesis Testing.
- ScienceDirect - Topics in Statistical Significance.
- Simply Psychology - What is Statistical Significance?